Bridging Diagnosis and Explainability using Multimodal Large Language Models: A Case Study on Retinal Disease Prediction From Fundus Images
Overview
As AI-driven medicine decision-making advances, deep learning models for retinal disease diagnosis have achieved impressive accuracy through training on large retinal fundus image datasets. However, these models often lack transparent explanations for their diagnostic outcomes, limiting their clinical applicability. The LUMI-EYE project explores using multimodal large language models (LLMs) to provide detailed, visually aligned natural language explanations for retinal disease diagnoses. This approach aims to make AI diagnostics understandable for different target audiences including ophthalmologists, medical students, and patients, thus supporting informed clinical decisions.
Methods Used
- Diagnosis Model: We use RETFound, a foundational model for retinal disease diagnosis, to generate accurate classifications for ocular diseases.
- Saliency Map Extraction: We apply Layer-wise Relevance Propagation (LRP) to generate saliency maps to highlight diagnostic-related areas in the images.
- Multimodal Dataset Creation: With expert support, we pair images with natural language explanations that describe key diagnostic features.
- LLM Fine-tuning: We fine-tune multimodal LLMs on our dataset with LoRA to build a system capable of generating explanations for retinal fundus images.
- Evaluation: We conduct human expert assessments along with quantitative and qualitative analyses to evaluate model-generated explanations.
Sample Results/Publications
We have completed an exploratory of the foundation diagnosis model and saliency map extraction. Initial zero-shot experiments with the most advanced multimodal LLMs (GPT4o, Llama3.2 Vision, LLaVA-Med) indicate their potential for aligning visual features with diagnostic context, as these models show promise in linking highlighted regions in saliency maps with pathology and anatomy context to explain the diagnosis. We are collaborating with ophthalmology experts to assess the generated explanations. Next, we plan to finalize our multimodal dataset and continue model training to enhance explainability in ocular diagnosis.
The extracted saliency maps look like this:
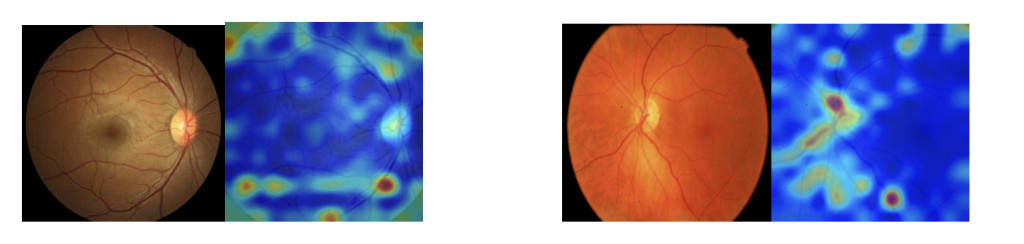
The overall pipeline (temporary) looks like this:
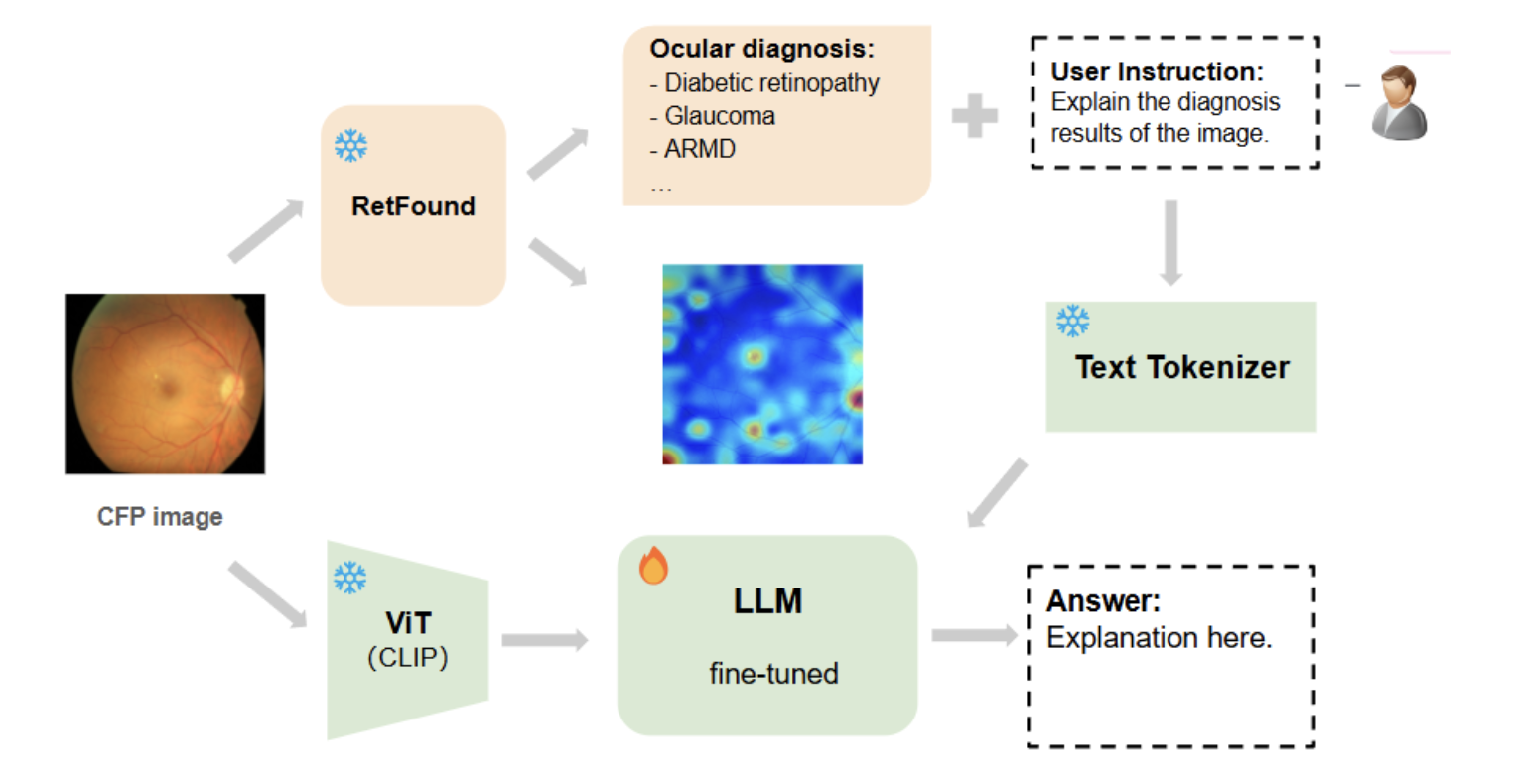
People involved
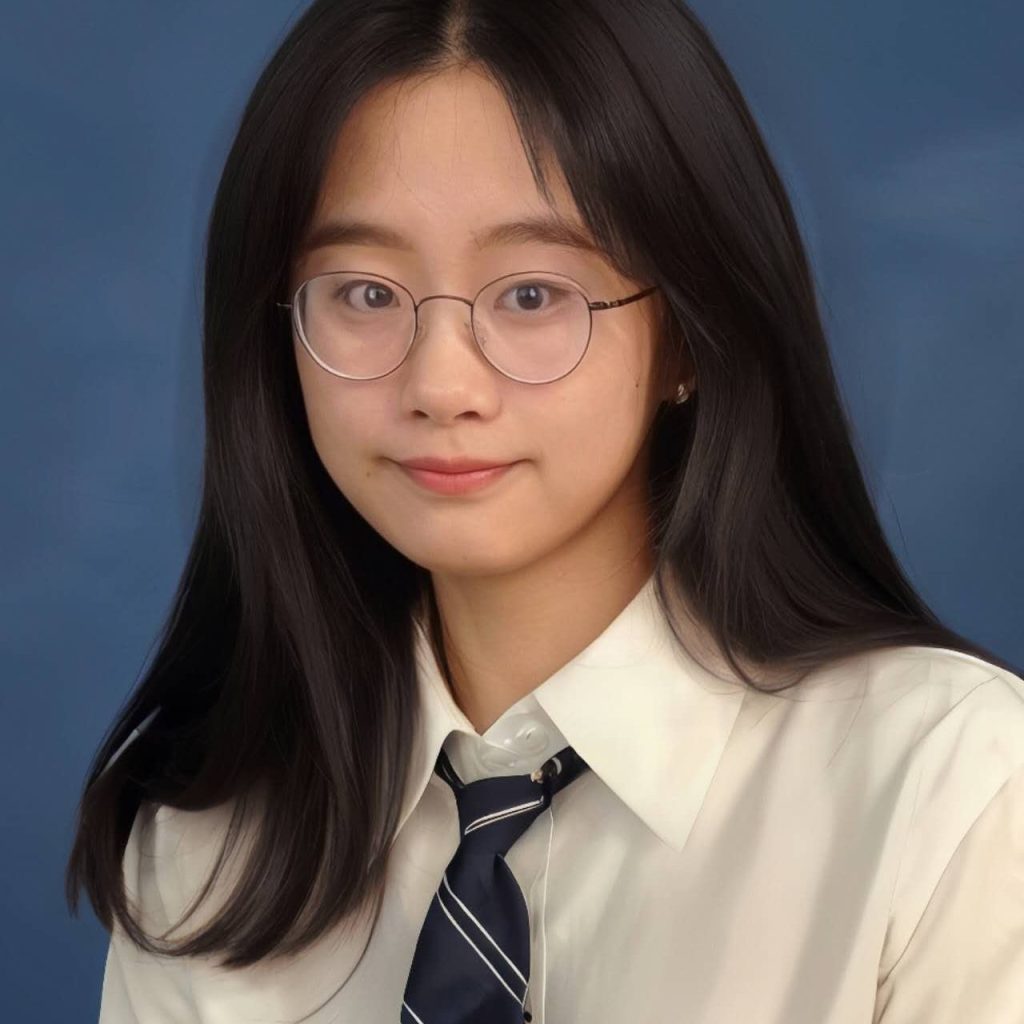
Yating Pan
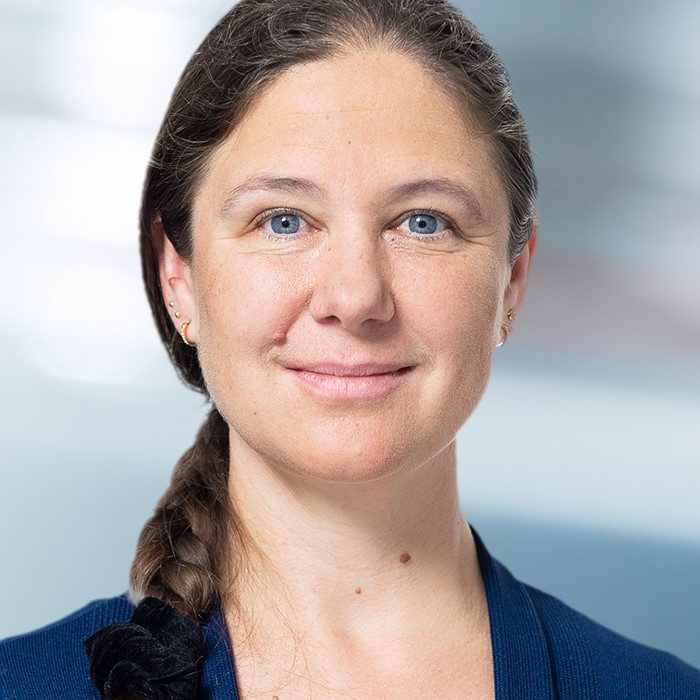
Janna Hastings
As well as Ophthalmology Experts.