Mental Health Ontologies: How we talk about mental health, and why it matters in the digital age
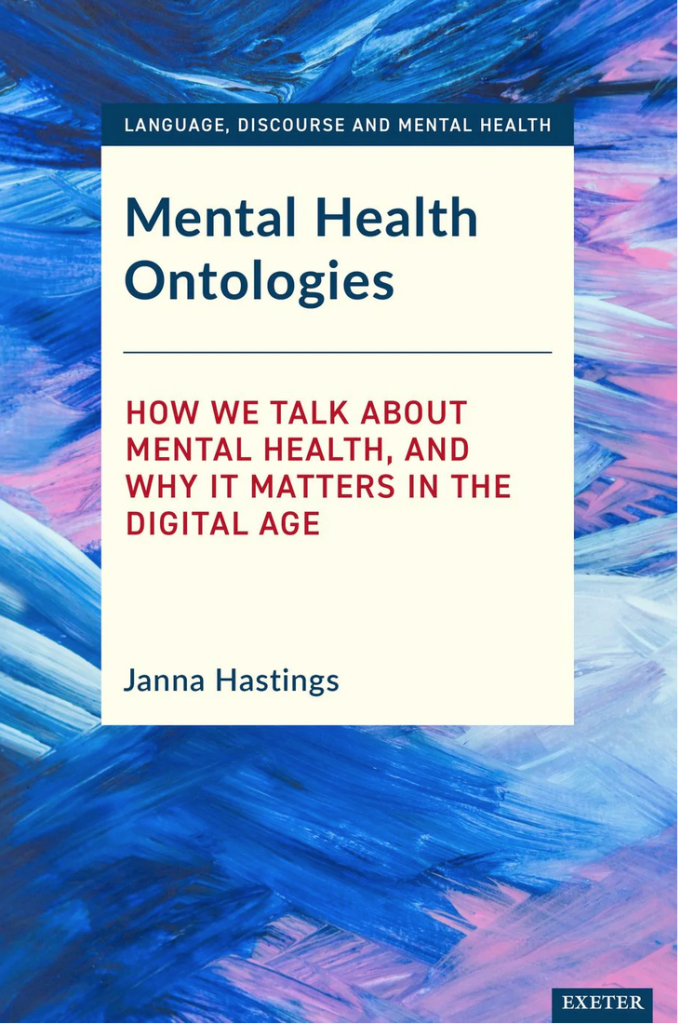
Mental health presents one of the defining public health challenges of our time. Debate and fragmentation around the nature of the entities that feature in the mental health domain divide resources and reduce progress. The way mental health is publicly discussed in the media has tangible effects, in terms of stigma, access to healthcare and resources, and private expectations of recovery. This book explores in detail the sorts of statements that are made about mental health in the media and public reporting of scientific research, grounding them in the wider context of the theoretical frameworks, assumptions and metaphors that they draw from. The author shows how a holistic understanding of the way that different aspects of mental illness are interrelated can be developed from evidence-based interpretation of the latest research findings. She offers some ideas about corrective, integrative approaches to discussing mental health-related matters publicly that may reduce the opposition between conceptualisations while still aiming to reduce stigma, shame and blame. In particular, she emphasises that discourse in the media needs to be anchored to an overview of all the research results across the field and argues that this could be achieved using new technological infrastructures.
Artificial Intelligence for Scientific Discovery
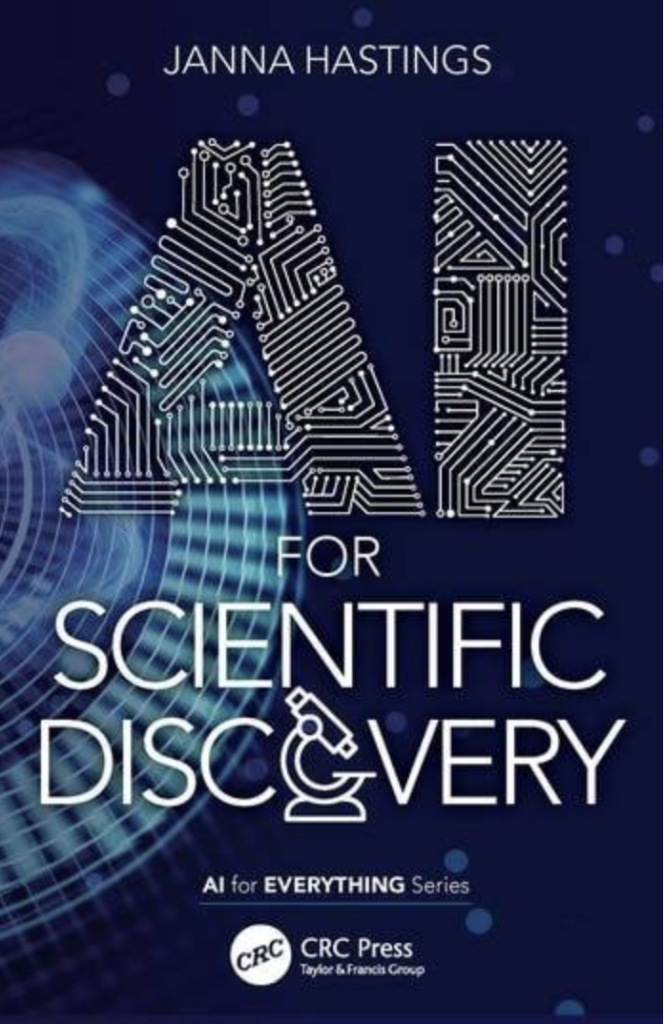
AI for Scientific Discovery provides an accessible introduction to the wide-ranging applications of artificial intelligence (AI) technologies in scientific research and discovery across the full breadth of scientific disciplines. AI technologies support discovery science in multiple ways. They support literature management and synthesis, allowing the wealth of what has already been discovered and reported on to be integrated and easily accessed. They play a central role in data analysis and interpretation in the context of what is called ‘data science’. AI is also helping to combat the reproducibility crisis in scientific research by underpinning the discovery process with AI-enabled standards and pipelines and supporting the management of large-scale data and knowledge resources so that they can be shared and integrated and serve as a background ‘knowledge ecosystem’ into which new discoveries can be embedded. However, there are limitations to what AI can achieve and its outputs can be biased and confounded and thus should not be blindly trusted. The latest generation of hybrid and ‘human-in-the-loop’ AI technologies have as their objective a balance between human inputs and insights and the power of number-crunching and statistical inference at a massive scale that AI technologies are best at.