Evaluating Text-to-Image Generated Photorealistic Images of Human Anatomy
Overview
Generative AI models have gained immense popularity and are used in many different contexts. However, issues like socio-cultural implications of the increasing presence of these images in our daily lives (e.g. deepfakes, misinformation, reinforcing biases and damaging body images) or the evaluation and comparison of photorelaistic images for e.g. medical applications (like medical education or surgical planning) needs to be examined. We develop an error classification system for annotating errors in AI-generated photorealistic images of humans and apply our method to a corpus of 240 images generated with three different models (DALL-E 3, Stable Diffusion XL and Stable Cascade) from a wide range of prompts. We found that DALL-E 3 performed consistently better than Stable Diffusion, however, the latter generated images reflecting more diversity in personal attributes.
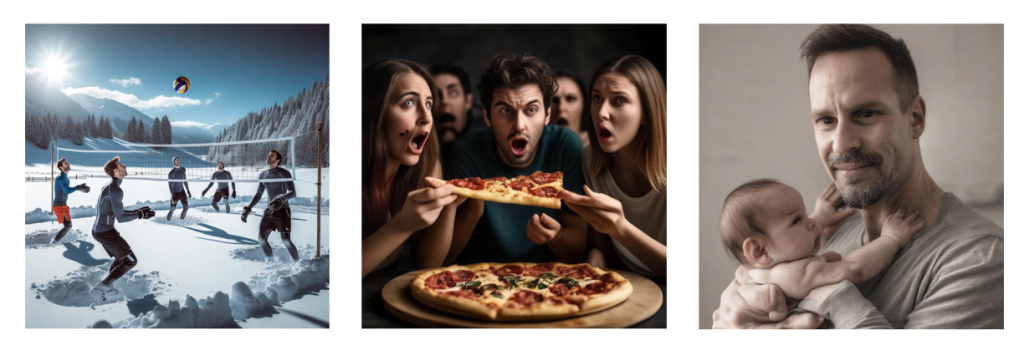
Methods used
We generated images based on prompts using three different models generative AI models: DALL-E 3, Stable Difussion XL and Stable Cascade. We iteratively developed an error classification system, which classifies 5 different types of errors in 5 different body parts and of 3 error severity levels. We used Krippendorf’s Alpha to asses the inter-annotator agreement of our four annotators, which we trained using a manual. We use the results of the evaluation to assign a cumulative score to each image to then compare the models itself as well as the prompts using standard statistics.
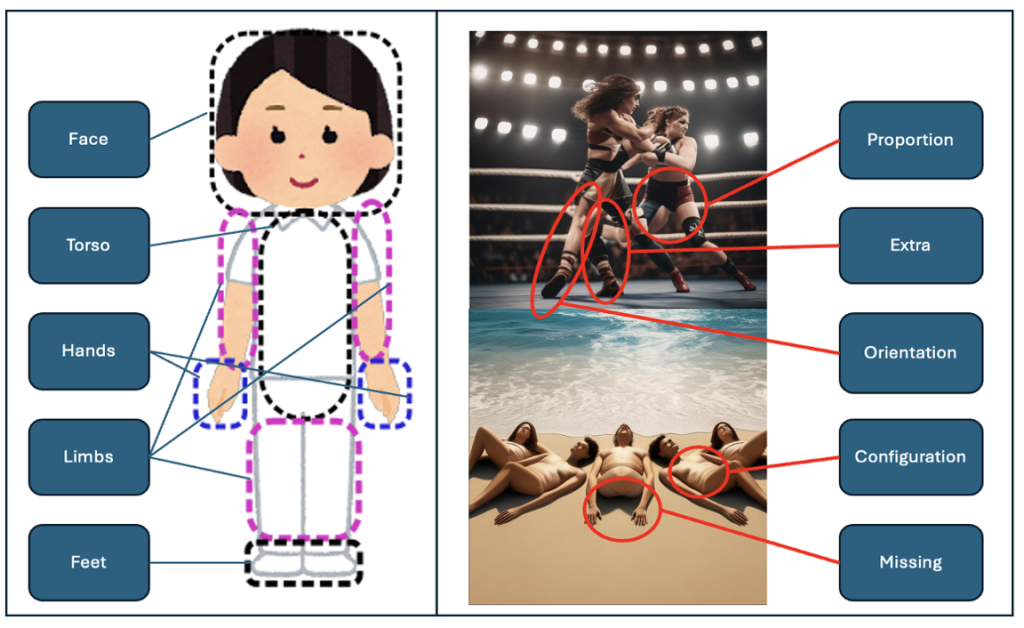
Sample results/Publication
We found that DALL-E overall achieves lower cumulative errors than Stable Diffusion XL and Stable Cascade. For all models we could observe that certain prompts are more difficult than others, as well as hands being the most error-prone body part.
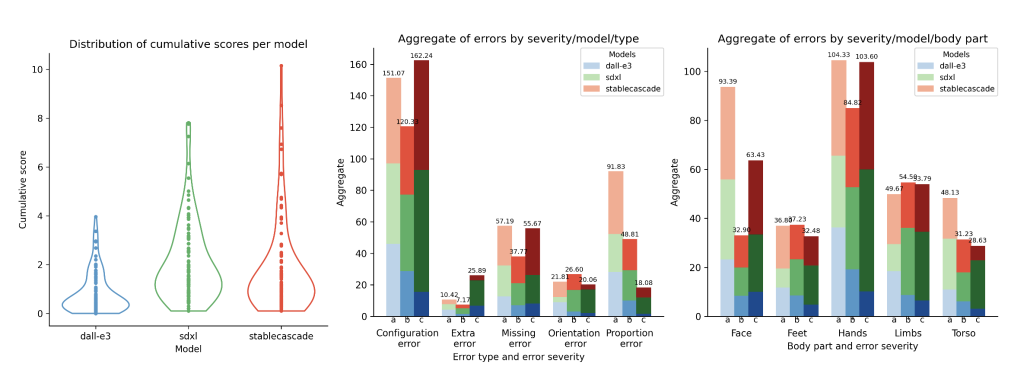
Find our GitHub Repository here.
People involed
As well as: Ann-Kathrin Kübler, Hatice Kübra Parmaksiz, Cheng Chen, Pablo Sebastián Bolaños Orozco, Soeren S. Lienkamp
/